Environment rather than character displacement explains call evolution in glassfrogs
- Colección Nacional Anfibios y Reptiles
- 5 oct 2023
- 43 Min. de lectura
Article in Evolution · December 2022 DOI: 10.1093/evolut/qpac041
5 authors, including:




Evolution, 2023, 77(2), 355–369 https://doi.org/10.1093/evolut/qpac041 Advance access publication 8 December 2022
Original Article
Environment rather than character displacement explains call evolution in glassfrogs
Angela M. Mendoza-Henao, PhD1,2,3, Kelly R. Zamudio, PhD4,5, Juan M. Guayasamin, PhD6,7, Moisés Escalona, PhD8, Gabriela Parra-Olea, PhD1 1Departamento de Zoología, Instituto de Biología, Universidad Nacional Autónoma de México, Mexico City, Mexico
2Posgrado en Ciencias Biológicas, Unidad de Posgrado, Ciudad Universitaria, Mexico City, Mexico 3Colecciones Biológicas, Instituto de Investigación de Recursos Biológicos Alexander von Humboldt, Boyacá, Colombia
4Department of Ecology and Evolutionary Biology, Cornell University, Ithaca, NY, United States 5Department of Integrative Biology, The University of Texas at Austin, Austin, Texas, United States 6Universidad San Francisco de Quito, Instituto Biósfera, Colegio de Ciencias Biológicas y Ambientales, Laboratorio de Biología Evolutiva, Quito, Ecuador 7Department of Biology, University of North Carolina at Chapel Hill, Chapel Hill, NC, United States 8Laboratório de Sistemática de Vertebrados, Pontifícia Universidade Católica do Rio Grande do Sul (PUCRS), Porto Alegre, Brasil Corresponding author: Departamento de Zoología, Instituto de Biología, Universidad Nacional Autónoma de México, Mexico City, Mexico. Email: gparra@ ib.unam.mx
Abstract The acoustic adaptation hypothesis (AAH) and ecological character displacement (ECD) are two potential mechanisms shaping call evolu- tion that can predict opposite trends for the differentiation of signals. Under AAH, signals evolve to minimize environmental degradation and maximize detection against background noise, predicting call homogenization in similar habitats due to environmental constraints on signals. In contrast, ECD predicts greater differences in call traits of closely related taxa in sympatry because of selection against acoustic interference. We used comparative phylogenetic analyses to test the strength of these two selective mechanisms on the evolution of advertisement calls in glassfrogs, a highly diverse family of neotropical anurans. We found that, overall, acoustic adaptation to the environ- ment may outweigh effects of species interactions. As expected under the AAH, temporal call parameters are correlated with vegetation density, but spectral call parameters had an unexpected inverse correlation with vegetation density, as well as an unexpected correlation with temperature. We detected call convergence among co-occurring species and also across multiple populations from the same species in different glassfrogs communities. Our results indicate that call convergence is common in glassfrogs, likely due to habitat filtering, while character displacement is relatively rare, suggesting that costs of signal similarity among related species may not drive divergent selection in all systems.
Keywords: trait evolution, advertisement calls, community phylogenetics, adaptation, bioacoustics, Centrolenidae
The relative importance of environmental and biotic selection in trait evolution is a key question for understanding the ori- gin and maintenance of biodiversity (Indermaur et al., 2010; Wellborn et al., 1996). Environmental selection operates on phenotypic variation of individuals in populations, yet inter- actions of species within communities also shape the selective landscape. Acoustic signals are the main type of communi- cation in several animal species (Bradbury & Vehrencamp, 2012) and they evolve in response to both environmental selection and biotic interactions (Chek et al., 2003; Endler, 1992; Krause, 1993; West-Eberhard, 1983). Acoustic signals are subject to temporal and spectral degradation, and atten- uation of calls in any particular environment depends on its sound frequency (pitch) (Wiley & Richards, 1982). The en- vironment can generate interference by distorting signals or impeding detection by the intended receiver (Goutte et al., 2013), affecting transmission fidelity, and generating subop- timal communication (acoustic interference). The acoustic adaptation hypothesis (AAH) posits that signals evolve to
minimize degradation in the environment and maximize de- tection against background noise (Ey & Fischer, 2009). In gen- eral, slow-paced and low-pitched signals should travel more effectively in closed habitats (such as rainforests), while fast- er-paced and higher-frequency signals should suffer greater at- tenuation and reverberation (Marten & Marler, 1977; Tobias et al., 2010; Wiley, 1991). In addition, the role of environ- mental temperature in acoustic communication has been well characterized in anurans (Gerhardt, 1994; Gerhardt & Huber, 2002). At the individual level, note rate and pulse rate (repe- titions in a period of time) increase as temperature increases, while call duration is reduced (Gerhardt & Huber, 2002; Wells & Schwartz, 2007; Köhler et al., 2017). These environmental- ly induced changes in call traits have consequences for signal recognition by conspecifics (Bosch, 2001; Gerhardt & Mudry, 1980); however, the effects of temperature on vocalizations at a macroevolutionary scale are less explored.
Ecological character displacement (ECD) is a process of trait evolution caused by exploitative competition with one or more sympatric species resulting in shifts in traits that affect resource use (Brown & Wilson, 1956; Krause, 1993). In par- ticular, reproductive character displacement (RCD, a form of ECD) causes greater differences in the reproductive traits (e.g., mating signals) of closely related taxa in sympatry than in allopatry because of selection in sympatry against costly mating mistakes (e.g., reduced viability or fitness of hybrids, wasted gametes, or missed mating opportunities) (Gerhardt & Huber, 2002; Grether et al., 2009; Lemmon, 2009; Pfennig & Pfennig, 2009; Wilkins et al., 2013; but see Meier et al., 2017; Seddon & Tobias, 2010). Because ECD and RCD promote differentiation in acoustic signals, and potentially reinforce reproductive isolation, both have been suggested as drivers of call variation in anurans (Garey et al., 2018; Villanueva- Rivera, 2014). However, recent studies have found reduced evidence of character displacement and greater clade-wide convergence in bird songs (e.g., Tobias et al., 2014a); also, it has been notoriously difficult to exclude alternative processes that might lead to patterns resembling ECD or RCD, such as variation in environmental factors, shifts in resource use, spe- cies sorting, or even random evolution (Stuart & Losos, 2013). While ECD predicts differentiation in acoustic emission due to potential competition for acoustic space, the AAH predicts call homogenization in habitats with similar acoustics due to environmental constraints on acoustic propagation and, thus, on signal transmission (Gasc et al., 2017), but the joint effect of these interacting drivers remains controversial.
Adaptations for mate recognition can be directly tested experimentally by comparing the performance (fitness) of syn- thetic calls and by measuring female choice (Bush et al., 2002; Klymus et al., 2012). However, for studies addressing mul- tiple interacting species, phylogenetic comparative methods of trait evolution also allow for the detection of adaptation through trait convergence in multiple species, and measuring the predictability of trait change, for instance, across environ- mental gradients (Losos & Glor, 2003). This broader compar- ative approach allows us to evaluate patterns of trait change in a historical framework across multiple species. By quantifying call heterogeneity and phylogenetic dis- tances of species at a given locality under a phylogenetic comparative framework, we can compare three scenarios to test the relative strength of AAH and ECD (Figure 1). In the first scenario (Scenario A, Figure 1), the differences among calls are independent of phylogenetic community heteroge- neity and the evolution of calls among species shows conver- gence due to other factors, such as environment or habitat filtering. This pattern is expected if species are optimizing acoustic space independent of phylogenetic relationships
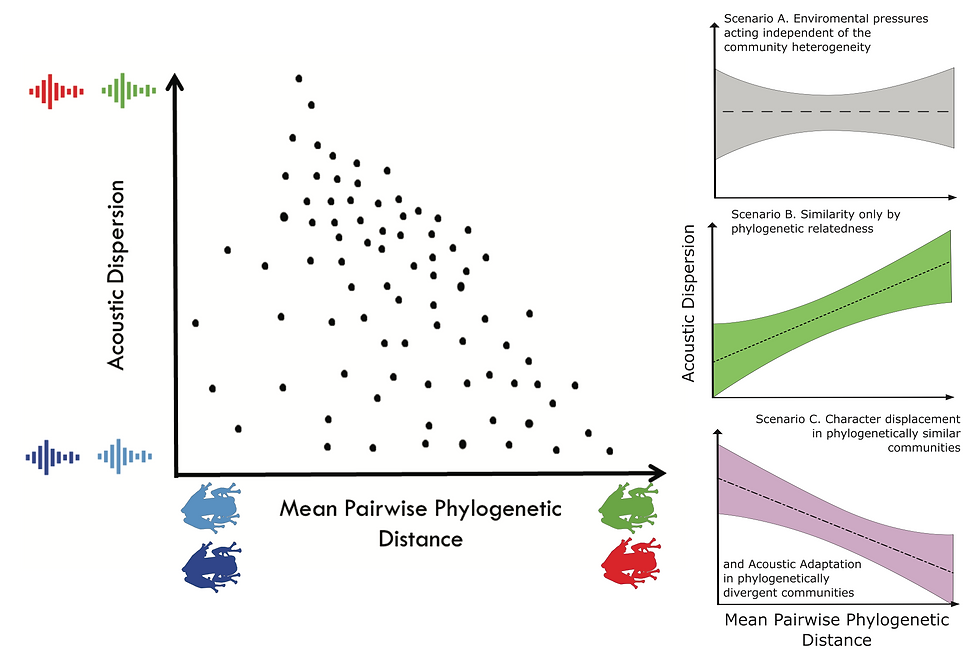
Figure 1. Expected evolution of advertisement calls under different scenarios: (A) Acoustic differentiation is unrelated to phylogenetic relationships of species within communities, and thus may be shaped by other factors such as environmental selection, (B) neutral call evolution is dominant, resulting in more divergent calls among more divergent species, and (C) character displacement is dominant in communities composed of close relatives, and acoustic adaptation predominates in phylogenetically divergent communities. The mean pairwise phylogenetic distance (MPD) is the mean of all pairwise branch lengths for a set of species in a community and the acoustic dispersion is the mean distance to the centroid in a phylogenetic PCA of calls from all localities where a species occurs. Species in communities with larger acoustic dispersion are exposed to more different calls than species with lower acoustic dispersion. Species in communities with high values of MPD live in heterogeneous communities (e.g., communities composed of species of different genera). For details see main text.
among community members, and if that optimization is dependent on abiotic environmental factors, then this sce- nario supports the AAH. In the second scenario (Scenario B, Figure 1), the divergence of calls is positively correlated with phylogenetic distance among community members. This pattern is expected if calls are evolving only due to neutral variation since species split from their common ancestor, or due to weak episodes of selection in varying directions, such that call evolution approximates pure neutral variation. This scenario does not support either ECD or AAH, it is the pattern expected if divergences in calls are entirely explained by divergence times among species. Finally, in the last sce- nario (Scenario C, Figure 1), species living in communities composed of very closely related taxa tend to have more dis- similar calls than expected by their phylogenetic relatedness (a signature of ECD), while species that live in communities composed by distantly related taxa show convergence in their calls, potentially because of acoustic adaptation. These three scenarios underscore the challenges of differentiating the relative roles of ECD and AAH. To do so, well requires having data on call traits for all species within repeated communities, knowledge of the phylogenetic relationships among species within communities, data on where species are in sympatry (to account for possible biotic interactions), and data on the environmental conditions affecting calls (abiotic selection). Phylogenetic comparative data allow us to test these associations at different levels of phylogenetic divergence, from populations to species, to infer the strength of ECD and AAH at different temporal scales.
Glassfrogs, in the family Centrolenidae, are a highly diverse Neotropical clade that includes 158 species (Guayasamin et al., 2020) and are an excellent group with which to test the relative roles of the acoustic adaptation and character dis- placement hypotheses in call evolution. Glassfrog species share ecological and reproductive traits: they all call at night, during the rainy season, and because they depend on stream habitats for reproduction, they experience noise-mask- ing produced by running water and waterfalls (Brunner & Guayasamin, 2020; Carvajal-Castro & Vargas-Salinas, 2016; Delia et al., 2017). A well-established phylogeny of glassfrogs (Castroviejo-Fisher et al., 2014; Guayasamin et al., 2008, 2020; Twomey et al., 2014) provides the framework for test- ing hypotheses about historical biogeography and diversifi- cation (Castroviejo-Fisher et al., 2014; Guayasamin et al., 2020; Hutter et al., 2013), patterns of phylogenetic diversity and endemism (Guayasamin et al., 2020; Mendoza & Arita, 2014), molecular evolution (Dugo-Cota et al., 2015), paren- tal care (Delia et al., 2017), and call evolution (Escalona et al., 2019). A recent detailed phylogeographic study of the widespread Hyalinobatrachium fleischmanni species complex (Mendoza et al., 2019) provides an excellent opportunity to test the same hypotheses about signal evolution among con- specific lineages at lower levels of divergence, such as deeply divergent lineages within a single species. In this study, we examine call trait evolution at the level of all species within the family Centrolenidae, and also at the intraspecific level, com- paring highly divergent lineages within Hyalinobatrachium fleischmanni.
Using comparative and community phylogenetic methods, we tested three hypotheses for the evolution of call signals: (1) Environmental conditions (i.e., temperature and vegetation density) act as drivers for call evolution in glassfrogs (due to Acoustic Adaptation), (2) glassfrog community composition shapes call evolution (through ECD and/or RCD), and (3) the strength of mechanisms leading to call differentiation will differ at different phylogenetic depths because different mechanisms operate from incipient species (deep conspecific lineages) to family levels. This phylogenetic approach allows us to investigate the relative roles of environmental and biotic selection (or lack thereof) on the evolution of acoustic signals at multiple evolutionary scales, to disentangle the selective context and mechanisms contributing to differentiation of this complex trait.
Materials and methods All subsequent analyses are based on two independent data- sets: the first focuses on interspecific comparisons across all Centrolenidae and specific clades in the family, the sec- ond focuses on intraspecific comparisons among deeply divergent lineages across the range of one species complex (Hyalinobatrachium fleischmanni). Unless otherwise noted, the same analyses were performed with both datasets.
Evolutionary patterns for call traits We reconstructed a phylogeny for glassfrogs, using all available sequences from GenBank plus additional sequences obtained for this study (Supplementary Appendix 1, Supplementary Table S1). The final dataset included 6304 bp of three mito- chondrial (12S (941 bp), 16S (871 bp), ND1 (961 bp) and seven nuclear loci (POMC (604 bp), BDNF (700 bp), C-MYC (406 bp), CXCR (356 bp), RAG (456 bp), SLC8A1 (542 bp), and SLC8A3 (467 bp) for 97 species in 12 glassfrog genera (ingroup; taxonomy sensu Guayasamin et al., 2009) and three species of Allophryne (outgroup). Sequences of each gene were aligned with ClustalW (Thompson et al., 1994) in Mega7 (Kumar et al., 2016); concatenated sequences were used in phylogenetic Bayesian reconstruction in BEAST2 (Bouckaert et al., 2014) using the CIPRES Science Gateway (Miller et al., 2010). We used Partition Finder 2.1.1 (Lanfear et al., 2016) to choose the best model of evolution for each gene. We then inferred ultrametric phylogenies using a log- normal relaxed-clock model and a Birth-Death speciation process (Drummond et al., 2006). We ran two independent analyses for 50 million generations, sampling trees and parameter values every 1,000 generations. Burn-in was set at 25%, and the first 12.5 million generations were discarded. A maximum clade credibility (MCC) tree was generated in TreeAnotator 1.8.4 (Bouckaert et al., 2014). For the H. fleis- chmanni complex, we reconstructed a phylogeographic topol- ogy for 16 populations sampled throughout the range of the species complex (Mendoza et al., 2019; Mendoza-Henao et al., 2020) and we extracted the MCC tree and 100 randomly sampled post burn-in trees for comparative analyses using the MCMCglmm function in the mulTree library (Guillerme & Healy, 2014).
We gathered all available glassfrog calls from sound collec- tions, and we supplemented the data set with contributions from colleagues, call descriptions available in literature, and our own recordings obtained in the field (Supplementary Appendix 2). Whenever possible we obtained up to 10 calls per species, to account for intraspecific variation. For com- parisons among conspecific lineages, we recorded a minimum of three calls, and a maximum of 10 for each locality sampled throughout the range of H. fleischmanni complex (Mendoza et al., 2019; Mendoza-Henao et al., 2020).
Within species, glassfrog calls are highly stereotyped and relatively simple (Guayasamin et al., 2020). The characteri- zation of calls is well-established and both spectral and tem- poral properties have been used to resolve taxonomic and evolutionary questions (see Köhler et al. [2017] and references therein). Therefore, for each species we obtained two spectral and four temporal call parameters: peak frequency (frequency at which the highest amplitude peak is found, also known as dominant frequency), frequency bandwidth (the difference between upper and lower-frequency bounds of notes, as mea- sured 6 dB below the peak frequency), note duration (length in milliseconds of a note, measured from beginning to end of the note), notes per call, pulse rate (number of pulses in a note minus 1, divided by the length of the note; with this formula, purely tonal calls always have a pulse rate of zero) and note rate (count of notes in a call minus 1, divided by the length of the call). We defined tonal notes as those consisting of a single frequency component at any time instant and pulsed notes as those composed by multiple short undividable sound units having a duration less than 50 ms, including spaced pulses with silent intervals (Köhler et al., 2017). Note series are separated from other such groups by periods of silence of longer duration than the inter-note intervals in a regular pat- tern. The first three parameters were also measured for the H. fleischmanni complex. Given that peak frequency is strongly dependent on body size (Escalona et al., 2019; Gingras et al., 2013; Tonini et al., 2020), we extracted the mean snout-vent length (SVL) for each species from the literature and from our own field measurements, and size-corrected the spec- tral attributes with the phyl.resid function (using the default options) in Phytools (Revell, 2012). The model estimates of the regression coefficients were the following: β = 5,644.2; x = −34.8. We measured call parameters from the wave format files in Raven Pro v.1.5 (Cornell Lab of Ornithology, Ithaca, NY, USA) with a Blackman window of 5 ms, an 80% overlap and a DFT of 1024.
The call metrics were reduced in a phylogenetic Principal Component Analysis implemented in Phytools (Revell, 2009). Before analysis, call parameters were standardized to a mean of zero and standard deviation of one. In addition, we performed two phylogenetic PCAs for only-temporal and only-spectral variables to evaluate potential drivers affecting each set of traits. We quantified the acoustic divergence as the Euclidean distance two first principal components for all combinations of pair of species of each phylogenetic Principal Component Analysis (PCA) (code available in github: https:// github.com/angymendozah/CallEvolGlassfrogs/). In the case of H. fleischmanni lineages, the Euclidean distances were con- structed between the calls of the H. fleischmanni from each locality against the call of all other glassfrog species (N = 13) reported at those localities, and we separated these values into two categories depending on whether the species co-occurred at each locality or not.
We estimated the best model of evolution for each call trait by comparing the AIC scores for five models with the fitCon- tinuous function in Geiger (Harmon et al., 2007): Brownian motion (BM), Pagel lambda (λ) Ornstein–Uhlenbeck (OU), Kappa (κ), or White Noise (WN). If call traits fit one of the first two, then the call trait follows a strict or relaxed Brownian motion without any particular trend detected. A fit to the OU model would lend support to stabilizing selection (as expected by the AAH), and a fit to Kappa model equal to zero would indicate a pattern of punctuated equilibrium, which would more closely support ECD because trait diver- gence is independent of branch length and might be enhanced during speciation events at the nodes of the tree. To explore the evolution of each acoustic trait at internal nodes in the tree, we reconstructed the evolutionary history of each trait using Bayesian MCMC with the function ancBayes in Phytools (Revell, 2012). We ran each MCMC chain for 10 million generations with 10% excluded as burn-in and we checked that the effective sample size for the call trait at each interior node was over 200 using the coda package (Plummer et al., 2006).
Testing the acoustic adaptation hypothesis We obtained georeferenced records to infer the distribu- tion for all glassfrog species included in this study from the database generated by Hutter et al. (2013), which was modified with updated species names and supplemented with recent data (final database comprised a total of 2,819 records, Supplementary Appendix 3). Our data comprise the most complete record of glassfrog species distributions assembled to date. Because our recordings were collected from many sources, we did not have temperature data at the time of recording for most of our species. Therefore, for each record, we extracted the environmental tempera- ture, estimated from the mean temperature of the wettest quarter (CHELSA BIO8, Karger & Zimmermann, 2019) as a proxy for the temperature during the rainy season, when glassfrog reproductive activity peaks. For H. fleischmanni lineages, we used the available air temperature registered in the metadata of the recording event. We also extracted veg- etation density, taken from the Enhanced Vegetation Index (EVI) proposed by the MODIS Land Discipline Group (Liu & Huete, 1995) and derived from aerial assessments of for- est canopy for both datasets, for this, we obtained the mean value per cell for 204 layers with monthly data between 2000 and 2017.
We tested for a correlation between environmental tempera- ture, vegetation density, and each acoustic trait by means of Markov chain Monte Carlo (MCMC) Sampler for multivar- iate generalized linear mixed models from the MCMCglmm package (Hadfield, 2010). MCMCglmm uses a MCMC esti- mation approach and accounts for non-independence among closely related species by including the degree of phyloge- netic relatedness in the variance–covariance matrix. We first attempted to fit our data to a linear trend; however, because of the left-skewed and zero-inflated distribution of some of our data and because of very large body size of a single spe- cies (Centrolene geckoidea) in comparison to all remaining glassfrogs, our data did not fit the assumptions required for a PGLS analysis, even after log-transformation. Therefore, we used the MCMCglmm analysis, which does not require the data to fit all PGLS assumptions. We included the effect of body size in our analysis for peak frequency, treating SVL as a second explanatory variable. Because vegetation density and air temperature are highly correlated (0.60 Pearson’s correla- tion coefficient, t = 7.342, df = 95, p-value <.001), each metric was included as a random effect in the MCMC model of the other.
Testing the character displacement hypothesis To test this hypothesis, we applied two approaches, one using species pairs, and the second using overall-community composition.
For the species pairs analysis, we created a presence–absence matrix on a 0.05 × 0.05 degree grid over the georeferenced records and we then estimated the overlap in distribution for each pair of species, calculated as the proportion of the smaller range that overlaps within the larger range (Chesser & Zink, 1994), using the lets.overlap function of the letsR package. We followed an approach similar to that used for bird communities (Tobias et al., 2014b) to test the direct impact of coexistence on call divergence correcting for common ancestry. To do so, we ran an MCMCglmm between call divergence (Euclidean distances in the PCAs) and coexistence (range overlap) including the phylo- genetic relationships among species in the variance–covariance matrix. Our analysis was performed with all pairwise coexis- tence and call difference values. We obtained multiple pairwise comparisons for each species, so we included species identity as a random effect (Tobias et al., 2014b). One caveat of our anal- yses is our choice of proxy for historical species interactions (Harmon et al., 2019). It is difficult to equate geographic overlap with true species interactions, even in areas with extensive sam- pling. In addition, although co-occurrence in the present day is a common proxy for species interactions, it does not necessarily imply long-term contact (Mayfield & Levine, 2010). Here we measured species co-occurrence by geographic overlap based on geo-referenced museum specimen records and we incorporated the best available information, including information on syntopy generated by our own field work or published data (Guayasamin et al., 2020; Hutter & Guayasamin, 2012; Mendoza-Henao et al., 2021). Although this approach is still far from perfect to assess the historical pattern of interactions among species (Losos & Glor, 2003), it does allow us to detect evolutionary patterns at large geographic scales more accurately than the conventional overlap of distributional polygons (Peterson et al., 2016).
For the overall-community analysis, we calculated commu- nity phylogenetic heterogeneity with the mean pairwise phylo- genetic distance (MPD), measured as the mean of all pairwise branch lengths for a group of species present in a community (Webb et al., 2002). The MPD, derived in the MetricTester pack- age (Miller et al., 2017), assesses if individual species coexist with closely related, distantly related, or a random set of species. The MPD is not inherently correlated with species richness and, therefore, does not confound our estimates of community phy- logenetic heterogeneity with site-specific species richness (Miller et al., 2017). For call divergence, we constructed an Acoustic Dispersion index for each species as a metric of the call het- erogeneity to which the species is exposed. To do so, we first measured the average distance to the centroid of all calls within each cell of the presence–absence matrix by calculating the Functional Dispersion index (Laliberté & Legendre, 2010) of the phylogenetic PCA of the calls. Then, we estimated the overall acoustic structure for each species by averaging the Functional Dispersion of all sites in which a particular species occurred, following the Qr-mode suggested by Arita et al. (2008). Thus, taxa in sympatry with species whose calls are very different will show higher Acoustic Dispersion, while those living with spe- cies having similar calls will show lower Acoustic Dispersion. We performed a MCMCglmm between the Acoustic Dispersion and the community phylogenetic heterogeneity per species, add- ing temperature and vegetation as random effects.
Testing mechanisms of selection at different phylogenetic scales To evaluate if call evolution in response to environmental and biotic selection occurs similarly at different phylogenetic levels, we used a deconstructive approach (Marquet et al., 2004). At the macroevolutionary scale, we performed anal- yses at the family and subfamily levels, and in four clades (nodes highlighted by white letters in Figure 2). For the H. fleischmanni complex, we analyzed all populations within the complex.
Finally, to test if the character displacement was evident only at the level of sister species, we extracted pairs of sis- ter species (N = 26) and divided those into two groups, one composed of pairs of species with range overlap (N = 7) and those with no range overlap (N = 19). These pairs of sister species with no range overlap were split into those separated geographically by a well-defined barrier without differences in environmental conditions between them (N = 8) and those living in localities with different environmental conditions (e.g., different altitudinal range) (N = 11). We pruned the phylogenetic tree to include one terminal per pair of sister species and the call divergence among these three groups was compared using a Phylogenetic ANOVA (Revell, 2012). Under an ECD scenario, we expect that pairs of sister species with range overlap will exhibit higher differences in calls than pairs of sister species with no range overlap.
Results Evolutionary patterns for call traits Our phylogenetic tree included 97 glassfrogs species for which we had advertisement call data (Supplementary Appendix 1, Supplementary Table S2.1). This includes representatives from all twelve genera and covers 61.3 % of species diver- sity in the family. The phylogeny is consistent with previous studies (Castroviejo-Fisher et al., 2014; Delia et al., 2017; Guayasamin et al., 2008, 2020; Twomey et al., 2014) and shows strong support for the monophyly of glassfrog genera and their relationships (posterior probabilities >.95 for most nodes, Figure 2).
The peak frequency of calls ranged from 2,713.2 Hz (Ikakogi tayrona) to 7,407.4 Hz (Chimerella corleone) with a mean value of 4,950.6 Hz. Centrolene sanchezi showed the shortest note duration (3.6 ms) and Centrolene condor the longest (601.0 ms). More than half of the species (N = 62) displayed only one note per call while the remaining (N = 35) displayed between two and nine notes. Forty-three species showed a tonal call (each note contains a single frequency component at any time instant) and the remaining 52 showed pulsed calls (each note contains a series of energy bursts sep- arated from each other by distinctly reduced amplitude). The pulse rate ranged from 19.8 pulses/s (Hyalinobatrachium tri- color) to 309.9 pulses/s (Sachatamia ilex).
Our distributional database showed that glassfrog species are present in localities with mean temperatures of wettest quarter ranging from 11.9 °C (Centrolene aff. buckelyi) to 25.7 °C (Hyalinobatrachium kawense) and with vegetation density (values for EVI spectral Index) ranging from 3,474.6 (Centrolene sabini, from high-elevation valleys in Peru) to 5,739.4 (Vitreorana castroviejoi, below 1,000 m in Cordillera de la Costa in Venezuela). The calls of H. fleischmanni indi- viduals showed no variation in the number of notes or note rate among 16 localities, but did exhibit variation in peak frequency (4,112.8–5,343.8 Hz), note duration (0.089– 0.159 ms), and frequency bandwidth (864.1–1,189.9 Hz). Complete call metrics, environmental values and body size for all species and for populations within H. fleischmanni
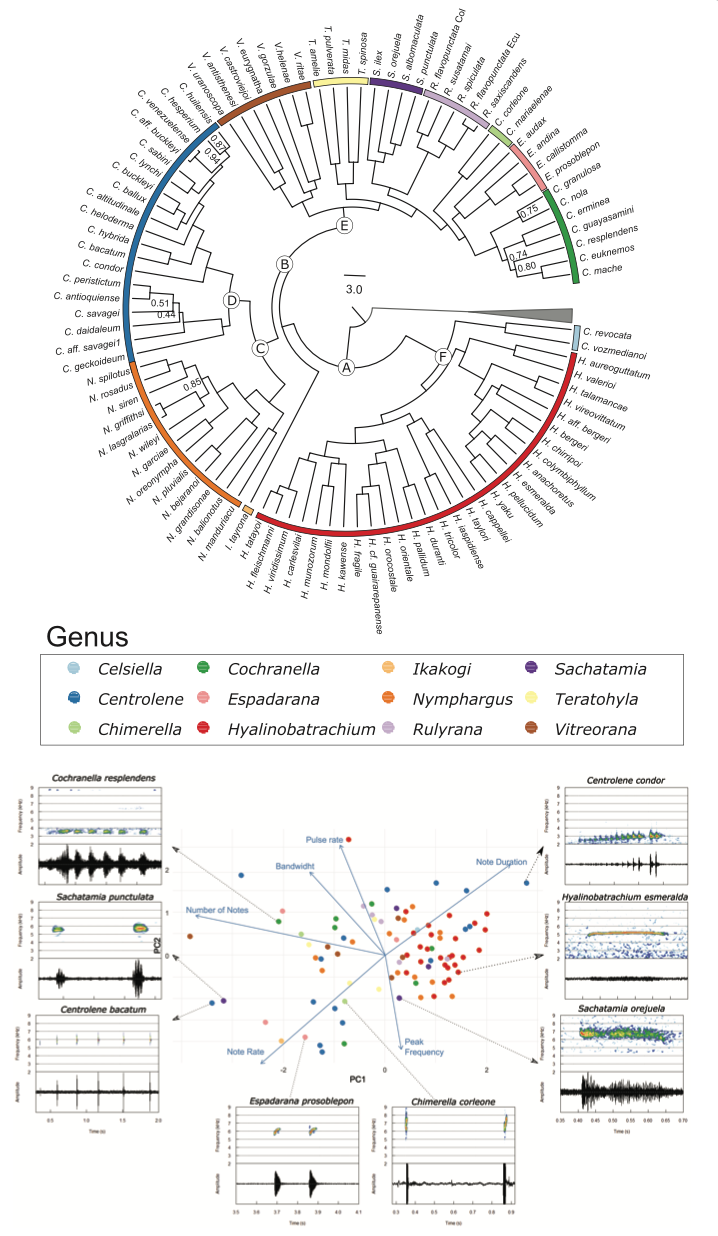
Figure 2. (A) Bayesian phylogenetic reconstruction of 97 glassfrog species based on DNA sequences from three mitochondrial (12S, 16S and ND1) and seven nuclear (POMC, BDNF, C-MYC, CXCR, RAG, SLC8A1, and SLC8A) markers (6304 bp). Allophrynidae species (gray triangle) were used as outgroup. Scale bar show mean calibration in million years. White circles identify the crown node of Centrolenidae and analyzed subclades. A = Centrolenidae, B = Centroleninae, C = Centrolene + Nymphargus, D = Centrolene, E = Cochranellini, F = Hyalinobatrachinae. Colors at the tips of the tree represent genera. Clade support < 0.95 is indicated by posterior probability values from Bayesian Inference. (B) Call variation among glassfrogs species, displayed with the phylogenetic PCA of six call parameters analyzed in this study. Insets are examples of spectrograms and sonograms for eight species with highly divergent calls. PeakFreq = Peak Frequency, BandW = Frequency Bandwidth, Nrate = Note rate, MNotes = Maximum number of notes, PR = Pulse rate, NDur = Note duration.
are provided in Supplementary Table S1. The first two com- ponents of the phylogenetic PCA for all call traits explained 53.5 % of the variance (Figure 2). Number of notes had the highest loading for the first component, while note rate, pulse rate, and peak frequency had higher loadings in the second component. The phylogenetic PCAs for all parameters as well as PCAs for only-spectral and only-temporal parameters are provided in Supplementary Tables S2.1, S2.2, and S2.3.
By comparing the evolutionary models that best fit call traits based on the AIC criterion (Table 1 and Supplementary Table S3), we found that Pagel’s lambda is the best-fit model of evolution for peak frequency, frequency bandwidth, note duration and the residuals of peak frequency against SVL, suggesting that those call traits follow a Brownian Motion model in a scenario with a relaxed phylogenetic signal (labil- ity to pure Brownian Motion given by 1 − lambda value). In contrast, Ornstein–Uhlenbeck (OU) was the best-fit model of evolution for note rate and pulse rate, suggesting that these parameters are constrained around an adaptive optimum over time. Pulse rate had the highest instantaneous rate of change (sigma square), while note duration and bandwidth had the lowest. Among populations within the H. fleischmanni com- plex, Pagel’s lambda was the best-fit model of evolution for peak frequency, OU model for note duration, and Brownian Motion for frequency bandwidth.
Finally, ancestral state reconstructions (Figure 3) revealed an evolutionary trend toward higher peak frequencies within all genera except Nymphargus (Clade F; Figure 3A). A few spe- cies showed independent changes from medium to low peak frequencies and from medium- to low-frequency bandwidth values. In all species of the subfamily Hyalinobatrachinae (Clade B), the number of notes is one, and thus the note rate is zero (Figure 3C and D); for Centroleninae (Clade D), we found multiple cases of evolution from low to higher number of notes (Figure 3C). Pulse rate was the trait with most variation among glassfrogs (Figure 3F); while some subclades of Hyalinobatrachium, Nymphargus, Teratohyla, and Vitreorana show relatively low change in pulse rate among species, the remaining clades in the family had highly variable pulse rates even between sister species. Ancestral reconstruction of size–frequency residuals is provided as Supplementary Figure S1.
Acoustic adaptation We found a correlation between vegetation density and tem- perature with some call parameters at multiple evolutionary levels in the glassfrog phylogeny, suggesting that the environ- ment plays an important role in call evolution. The general- ized linear mixed models (MCMCglmm), accounting for the statistical non-independence of closely related taxa and body size (Figure 4A, details in Supplementary Table S4), detected a positive correlation between peak frequency and both environmental variables at almost all levels. The exceptions to this correlation were within the Cochranellini tribe, were we found no correlation with either environmental variable, and within the subfamily Hyalinobatrachinae, where a mar- ginal positive correlation was found only between peak fre- quency and temperature. Note rate was positively correlated with temperature at all scales, except for the Cochranellini tribe. The number of notes was negatively correlated with vegetation density at the family scale, within the subfamily Centroleninae, and for the Centrolene + Nymphargus group. Frequency bandwidth, pulse rate, and note duration were not correlated to either environmental variable. For populations within the H. fleischmanni complex, none of the call parame- ters were correlated to the either environmental variable.
Character displacement versus acoustic adaptation In the species pairs analysis, we found a convergence of calls in species with higher range-overlap, a pattern opposite that expected under character displacement. A negative correla- tion of spectral parameters and range-overlap was found for the entire family (post.mean = −0.198, 95% confidence interval [CI] = −0.395 to −0.003, pMCMC = 0.049) and for the Centroleninae subfamily (post.mean = −0.259, 95% CI = −0.500 to −0.014, pMCMC = 0.035), while accounting for the relatedness of taxa. Details of these tests are provided in Supplementary Table S4.2.
In the overall-community analysis (Figure 4B), the phylo- genetic heterogeneity of the community was not correlated with the acoustic dispersion for spectral parameters (sce- nario A, Figure 1) at the family level and for almost all sub- clades. However, we found a negative correlation between phylogenetic heterogeneity of the community and acoustic
Table 1. Best fit model of evolution for each acoustic trait in glassfrogs.
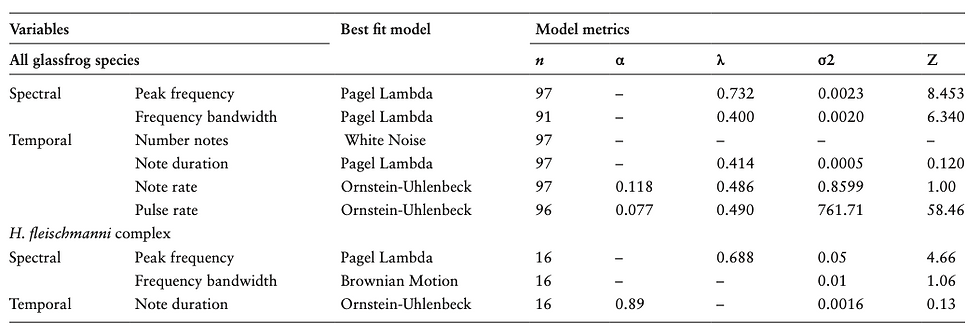
Note. α = constraint parameter, n = number of tips analyzed, λ = Pagel Lambda, σ2 = rate parameter and Z = starting value.
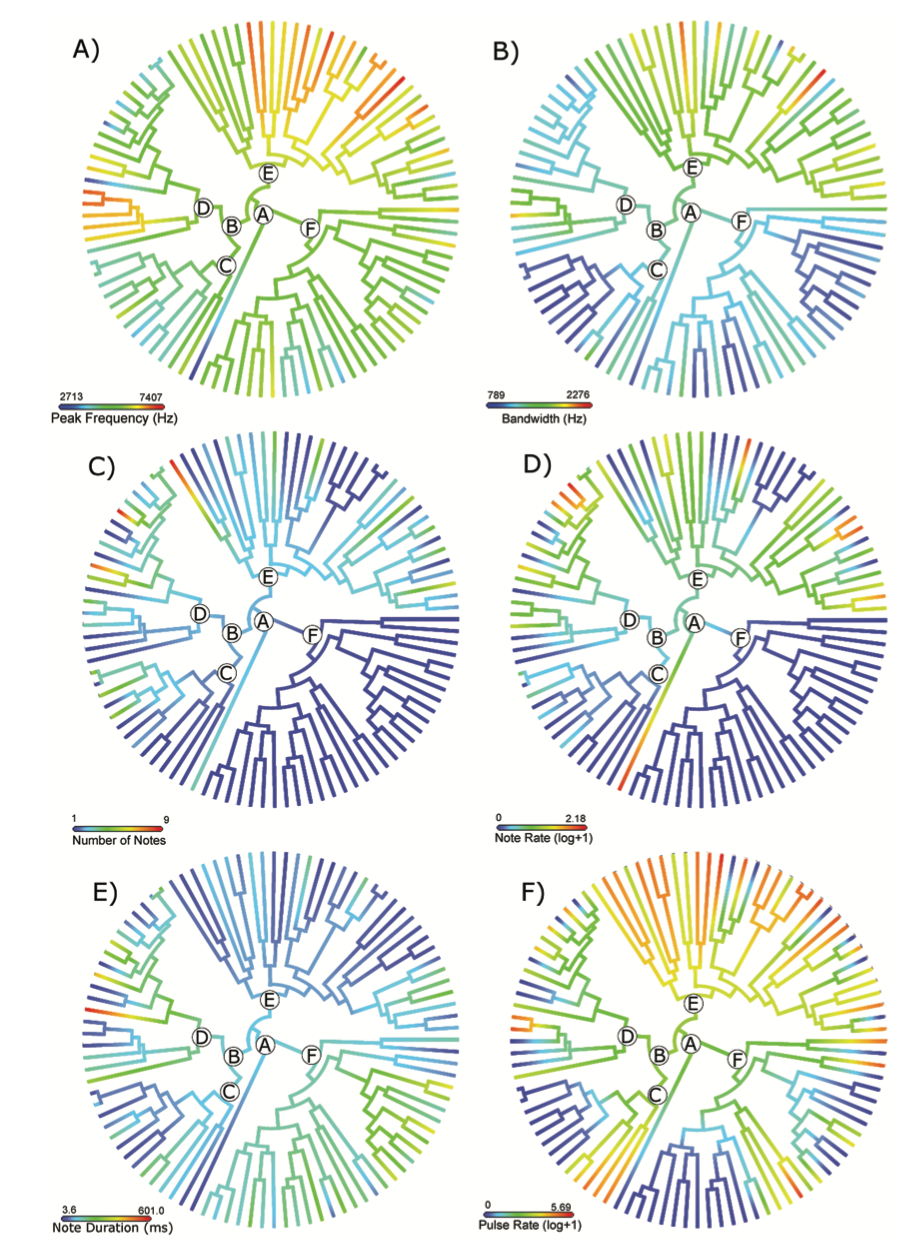
Figure 3. Ancestral state reconstruction of six call traits in glassfrogs. (A) Peak frequency (Hz), (B) frequency bandwidth (Hz), (C) number of notes, (D) note rate, (E) note duration (ms), and (F) pulse rate. Color legend below each tree denotes minimum and maximum values for each trait. Letters at nodes identify the same clades in Figure 3A. Trees for these analyses were pruned depending on the availability of trait data for each species.
dispersion in the analysis including only temporal param- eters in the PCA restricted to Centroleninae (post.mean = −0.258, 95% CI = −0.493 to −0.0037, pMCMC = 0.039), indicating that in this subclade character displacement may be occurring in communities with closely related species (scenario C, Figure 1).
Evolution (2023), Vol. 77, No. 2

Figure 4. Summary of the results for acoustic adaptation (A) and combined acoustic adaptation/character displacement (B) at different phylogenetic levels. Horizontal bar represents posterior mean (slope) values and their 95% compatibility intervals (CI) for each test through Bayesian mixed-model approach. Line colors in Figure 4A represents vegetation (green) and temperature (red) and line colors in Figure 4B represents overall call (brown), only- temporal (dark cyan) and, only-spectral (violet). Overlap of horizontal line with the vertical black dashed line indicates non-significant correlation among variables. A–F letters shows the position of each clade in the Figure 2A tree. Details of the analyses are provided in Supplementary Table S4.
Character displacement of sister species and deep conspecific lineages The lowest values for call divergences were found in four of the eight allopatric sister-species in similar environments and the highest call divergences were observed in sister-species inhabit- ing different environments (Figure 5A), suggesting that the envi- ronment might exert more pressure on calls of sister-species than the community call composition. However, the phylogenetic ANOVA did not detect significant differences among call diver- gence of pairs of sister species of three categories based on range-overlap and environment similarity (allopatric species in different environments vs. species with range-overlap: t-val = −0.005, p = 0.997; allopatric species in different environments vs. allopatric species in similar environments: t-val = −1.798, p = 0.303; species with range overlap vs. allopatric species in similar environments: t-val = −1.610, p = 0.303).
In the MCMCglmm analysis of coexistence versus call divergence for populations, the calls of different populations of the H. fleischmanni complex were more similar to those of sympatric glassfrog species (post.mean = −0.110, 95% CI = −0.215 to −0.008, pMCMC = 0.036), suggesting a con- vergence of the calls at this evolutionary scale (Figure 5B). The Euclidean distance between calls of H. fleischmanni and other glassfrog species was shorter when they occurred at the same locality. The distances of H. fleischmanni calls and H. collymbiphyllum and H. valerioi calls appear to be higher when they are registered in the same locality (Figure 5B), but the number of calls sampled was too low to perform a statis- tical comparison restricted to only those species.
Discussion The goal of our study was to address whether glassfrog signals have evolved primarily in response to environmental or biotic selective pressures, and to explore the role of these selective mechanisms at different evolutionary scales. Our results partially contradict the patterns expected under both the AAH and ECD.
Acoustic adaptation If the advertisement calls of glassfrogs follow the AAH, we expect spectral parameters to change with vegetation density (species in denser vegetation will exhibit low-pitched calls), and temporal parameters to change both with vegetation density (species in denser vegetation will exhibit lower pulse rate and longer note duration) and temperature (species at warmer sites will have higher note rate and pulse rate and shorter note duration) (Ey & Fischer, 2009). However, our results for spectral parameters show the opposite pattern than expected under the AAH. Glassfrog species living in locali- ties with denser vegetation have significantly higher peak fre- quencies, which, in theory, will suffer greater attenuation and reverberation under such circumstances (Figure 4A) (Wiley & Richards, 1982). The Acoustic Adaptation predictions were also not supported for call variation within the H. fleis- chmanni complex. We predicted that low-pitched and longer (slower) calls would be more common at denser vegetation sites to increase the likelihood of their signals reaching the intended receiver (Ey & Fischer, 2009); however, none of the call parameters of H. fleischmanni showed any correlation with vegetation density (Figure 4A).
Some predictions of the AAH were supported for temporal parameters. We found that, for some clades, species living in habitats with denser vegetation have fewer number of notes, as expected under selection for efficiency of sound transmis- sion (Figure 4A). A lower repetition rate reduces overlap with reverberated waves in closed habitats (Ey & Fischer, 2009). The fact that temporal call parameters show this association with potential environmental drivers is corroborated by our finding that temporal traits best fit an Ornstein–Uhlenbeck model, indicating that these parameters are constrained around an adaptive optimum over time (i.e., stabilizing selec- tion (Hansen, 1997; but see Cooper et al., 2016). In addition, the temporal parameters exhibit the highest evolutionary rates (sigma square), possibly because they are responding to selection in relation to sound transmission efficiency, in
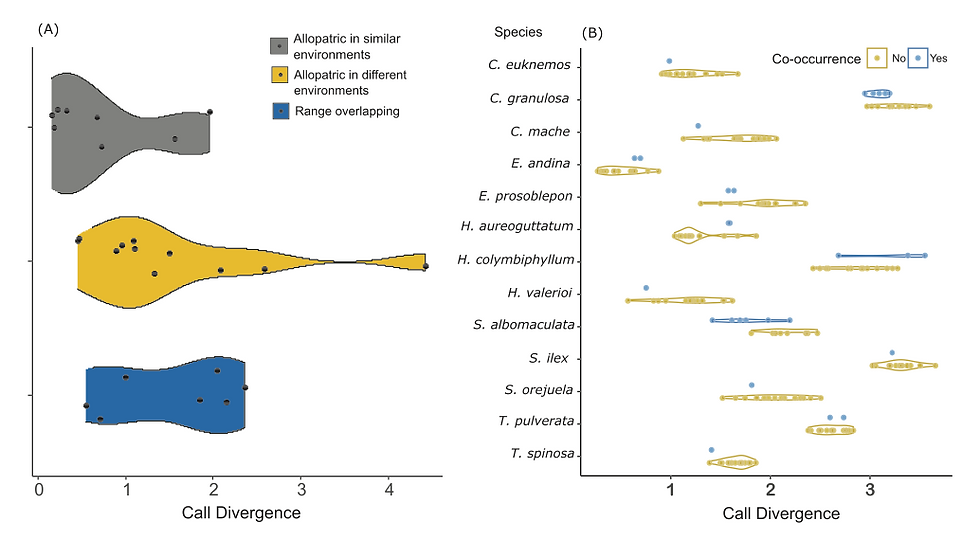
Figure 5. Violin plot of call divergence values between pairs of species. (A) Call divergence for 26 pairs of sister species across three categories: Allopatric species in similar environments (gray), allopatric species in different environments and (yellow), and sister species with range overlap (blue). (B) Euclidean distances of the call of Hyalinobatrachium fleischmanni complex against each glassfrog species in localities sympatry (blue) and allopatry (yellow).
addition to female selection (Ryan & Keddy-Hector, 1992). For anuran calls, it is not surprising that temporal parame- ters are more subject to environmental selection than spec- tral parameters (Gerhardt & Mudry, 1980), which are more constrained by morphology (Escalona et al., 2019; Tonini et al., 2020).
Although we found an impact of vegetation on the evo- lution of some temporal call parameters as predicted by the AAH, spectral parameters showed an opposite effect, indi- cating that the correlation of those traits to vegetation den- sity may be due to different mechanisms. Previous studies of the AAH in anurans using different measures of vegetation (open vs. closed, macrohabitat features, canopy coverage) have found contradictory results (Bosch & De la Riva, 2004; Goutte et al., 2018; Zimmerman, 1983). One study at the intraspecific level reported a strong effect of habitat structure on temporal call parameters among individuals (Ziegler et al., 2016). These contradictory results indicate that the selec- tive mechanisms linking habitat structure to the evolution of acoustic signals in other taxa may not be entirely transferable to frogs (Zimmerman, 1983).
We also did not find the expected relationships between temperature and call parameters in our interspecific com- parisons. Typically, temporal parameters but not spectral parameters of calls increase with temperature (Gerhardt & Huber, 2002; Ziegler et al., 2016), yet we found that species with calls at higher peak frequencies inhabit warmer regions (after accounting for the effect of shared ancestry, body size, and vegetation density). In addition, note rate (but not pulse rate nor note duration) was positively related to temperature, such that species living in warmer conditions have faster calls. The calls of the H. fleischmanni complex do not follow the common trend for anurans, warmer sites do not show shorter (faster) note durations (Figure 4A). These results indicate that air temperature has the expected impact on the advertisement calls of glassfrogs only at macro-evolutionary levels (i.e., gen- era, family) but not for close related lineages.
In summary, air temperature and vegetation density have a different effect than expected as drivers of call evolution for spectral parameters and for temporal parameters among glassfrogs species. What might be the cause of this pattern? If topographic heterogeneity drives stream noise, we should expect higher peak frequencies in species living in steeper terrains (probably at higher elevations) to avoid noise mask- ing. However, high elevation species (at colder sites with less vegetation) call at the lowest peak frequencies (Figure 4A), and no relation was found between peak frequency and rug- ged terrains (p = .05; β = −0.775; R2 = 0.053; see details in Supplementary Appendix 4); therefore, peak frequencies must be responding to a different factor, such as body size. Peak frequency is strongly related to body size (Augusto-Alves et al., 2021; Escalona et al., 2019; Goutte et al., 2016; Tonini et al., 2020), thus selection for size differences in anurans at different temperatures that are independent of sound trans- mission properties (e.g., Bergmann’s Rule) might be the pri- mary selective mechanism (Ashton, 2002; Bergmann, 1847; Gouveia et al., 2013; Ziegler et al., 2011). However, we found a weak relation between body size and temperature (post.mean = −0.040, l-95% CI = −0.083 to 0.001, pMCMC = 0.058), but a significant relationship of peak frequency and temperature, even after accounting for the effect of body size (Supplementary Table S2), indicating that this trend is not a secondary consequence of body size.
An increase in peak frequency (and in frequency prefer- ences) with temperature has formerly been reported in other anurans (Humfeld & Grunert, 2015; Meenderink et al., 2010; Narins & Meenderink, 2014), and this correlation has been associated with the temperature-dependent signal perception of one of the two hearing organs in the anuran inner ear, the amphibian papilla (Mohneke & Schneider, 1979; Narins & Meenderink, 2014). If the inner-ear of females plays a role in the evolution of spectral traits, frequency preferences (and selection) could be positively correlated to tempera- ture (Humfeld & Grunert, 2015; Meenderink et al., 2010; Narins & Meenderink, 2014). Future studies on inner-ear anatomy and function are needed to test the possible mech- anisms underlying the relationship between call pitch and temperature.
Character displacement
If community composition acts as a driver for call evolution among glassfrog species, we would expect differences in spe- cies calls to be greater when their range overlap is higher, but in most cases, advertisement calls from glassfrogs exhibit the exact opposite pattern. We found call convergence in species pairs with higher range overlap for all Centrolenidae, for the subfamily Centroleninae, and for comparisons among H. fleis- chmanni lineages (Figure 4B, Supplementary Table S3). The convergence of call parameters has also been observed in the acoustic signals of Amazonian bird communities and in acous- tic signaling of coexisting lineages of ovenbirds (Furnariidae) (Tobias et al., 2014a, 2014b). Therefore, our results align with a growing body of work suggesting that divergent forms of character displacement can be outweighed at macroevolu- tionary scales by either non-divergence, or even convergence (Tobias et al., 2014a). This convergence usually is attributed to habitat similarity (Cardoso & Price, 2010) and use of the same optimal resource, often referred to as “habitat filtering” (Webb et al., 2002). The higher similarity in calls of species with high range overlap supports the hypothesis that environmental fac- tors are driving the species in each locality toward an acoustic optimum. In this case, the call of all species in a given com- munity would be constrained by vegetation density and air temperature and, likely, other factors we did not test here.
One of the assumptions of character displacement is that extreme convergence of calls could have disadvantages for species in the community (Gerhardt & Huber, 2002; but see Meier et al., 2017). However, evidence from birds suggests that the evolutionary forces driving divergence in animal signals are weaker than is typically assumed (Seddon & Tobias, 2010; Tobias et al., 2014a). Species with convergent calls could min- imize competition through differences in other behavioral and ecological strategies. Glassfrogs share the same macrohabitat, they all call at night from riparian vegetation; thus, considering the close proximity to streams, they experience noise-masking for low frequencies by flowing water. To avoid this background noise, glassfrogs produce high-frequency calls, and in extremely noisy environments (i.e., Andean streams), selection for conver- gent evolution could be even higher. At a finer scale, glassfrog species show some differences in reproductive behaviors that might reduce acoustic niche overlap; for example, species have been observed reproducing at different times during the rainy season (Hutter & Guayasamin, 2012; Salgado & Guayasamin, 2018) or spatially segregating in different nearby streams to fur- ther avoid sound interference (Blair & Doan, 2009; Gottsberger & Gruber, 2004; Hutter & Guayasamin, 2012).
Considering that our measures of vegetation density and temperature were derived from satellite images and interpo- lated data from weather stations, our study does not allow us to examine small-scale differences in abiotic parameters. Therefore, a corroboration of the findings presented here will require a detailed study on the natural history, microhabitat use, and reproductive timing among species with overlapping ranges.
Because competition can arise when species have very sim- ilar signals due to recent shared ancestry (Stuart & Losos, 2013) studies of RCD generally focus on sister species pairs (Grossenbacher & Whittall, 2011; Höbel & Gerhardt, 2003; Jansen et al., 2016; Lemmon, 2009). If RCD plays a role in call divergence during speciation, then we predict that allo- patric sister species without differences in their environmen- tal conditions will not experience divergence in traits related to reproductive isolation (Mayr, 1942). The phylogenetic ANOVA for call divergence in species with different distribu- tions and same habitat types showed no significant differences among groups. However, when we compare the pattern in call divergence of sister species belonging to our three categories, we did observe the lowest divergence values for allopatric pairs of species (Figure 5A) and the highest divergence val- ues for sister species experiencing geographic isolation with environmental divergence (e.g., living at different elevations, Supplementary Figure S2). This indicates that environmental divergence might exert higher selection for differences among advertisement calls than interspecific competition for acoustic space. If that is the case, further studies discriminating how speciation mode and environment interact to drive call diver- gence will require a rigorous evaluation of niche divergence at macrohabitat and microhabitat levels.
Integrating acoustic adaptation and character displacement at different phylogenetic depths Our results indicate that the call of all species in any given community is associated with vegetation density (which may be shaping some temporal parameters) and in some cases with air temperature (in the case of spectral and some tempo- ral parameters) and these factors promote trait convergence among species at the same locality. This pattern was detected between species and in conspecific lineages. Taking all evi- dence together, we find that differences in environmental conditions likely shape call divergence between pairs of sister species more than reproductive or ECD.
Call evolution in response to selective pressures may be evident at some levels and not others because selection might depend on the divergence among species. Comparing the community results in our deconstructive approach (Figure 4B, Supplementary Table S4.3), we found that patterns in call divergence differ substantially among and within clades. In most cases, we found no correlation of call divergence and community heterogeneity (scenario A; Figure 1) where the dif- ferentiation is not a by-product of neutral evolution and com- munity composition is also not driving call divergences among species, so environmental selective mechanisms are acting independently of community heterogeneity. However, the analysis for Centroleninae subfamily showed a negative correlation of call divergence and community heterogeneity, indicating that for species in this subclade, communities composed of closely related species experience character displacement while heterogeneous communities experience trait convergence due to habitat filtering (supporting the result obtained in the pairwise species analysis; Scenario 1C, Figure 1). Therefore, different subclades respond in different ways to selective pressures, and by decomposing the patterns into smaller subsets within the family, we obtain better insights into the mechanisms that might underlie trait changes. Those differences might be related to the overall variation in call traits, which is higher for Centroleninae than for Hyalinobatrachinae (Figure 3); notably, Centroleninae also showed a higher impact of envi- ronment on call evolution (Figure 4A). These results, along with the fact that H. fleischmanni populations show higher call similarity when in sympatry rather than in allopatry with other Hyalinobatrachium species, corroborates the hypothesis that character displacement occurs only in a few cases and is primarily detected for the most closely related species (Brown & Wilson, 1956). An important caveat is that other non-Cen- trolenidae frogs and sound producing insects are also present in the localities where glassfrogs communicate, and may con- strain the available window for signal divergence (Wilkins et al., 2013). Further studies of competition including the entire acoustic community and potential constraints on signal diver- gence in glassfrogs might help explain some of our findings.
Overall, our findings indicate that evolution of glassfrog acoustic signals responds more frequently to environmental selection, although the magnitude of these effects varies across multiple phylogenetic levels and for different clades. Also, con- gruent with findings in other terrestrial vertebrates (Seddon & Tobias, 2010; Tobias et al., 2014b), by including historical information in analyses of species composition, we support the hypothesis that character displacement is not a common mech- anism for evolution of anurans acoustic signals. Therefore, fur- ther research on acoustic signal evolution in frogs and other groups should not assume that the conventional predictions of AAH and ECD are applicable in every taxon.
Supplementary material Supplementary material is available online at Evolution (https://academic.oup.com/evolut/qpac041) Data availability Call descriptions and list of georeferenced localities used in this article are deposited on supplementary material and in dryad repository (https://doi.org/10.5061/dryad.c2fqz61cp). Phylogenetic tree nexus file is deposited on TreeBASE. Author contributions A.M.M.H. and G.P.O. designed the study; A.M.M.H. per- formed research; A.M.M.H., J.M.G., and M.E. contributed new reagents/analytic tools; A.M.M.H. and K.R.Z. analyzed data; A.M.M.H., K.R.Z., J.M.G., M.E., and G.P.O. wrote the paper. Funding statement A.M.M. was supported by Consejo Nacional de Ciencia y Tecnología fellowship 416922 through Posgrado de Ciencias Biológicas of the Universidad Nacional Autónoma de México (UNAM). M.E.S. was supported by Coordenação de aperfeiçoamento de pessoal de nivel su- perior (process number 1578956) and Conselho Nacional de Desenvolvimento Científico e Tecnológico (process number 140815/2018-0). This project was financially supported by Programa de Apoyo a Proyectos de Investigación e Innovación Tecnológica-DGAPA (UNAM) project 203617, the Rufford Foundation (Rufford Small Grant reference 18423-1), and the Universidad San Francisco de Quito (Collaboration Grants 11164, 16871). Conflict of interest: The authors declare no conflict of in- terest.
Acknowledgments We thank the Macaulay Library of Natural Sounds at the Cornell Laboratory of Ornithology, Fonoteca Zoológica at the Museo Nacional de Ciencias Naturales de Madrid (Fonozoo), Colección de sonidos ambientales of the Instituto Alexander von Humboldt, Fonoteca Neotropical Jacques Vielliard at the University of Campinas, M. Rada, S. Duarte, E. Barona, J. Delia, A. Acosta-Galvis, S. Castroviejo-Fisher, L. Ochoa, C. Barrio-Amorós, A. Catenazzi, R. Figueroa, O. Hernandez, A. Salgado, A. Fouquet, C. R. Hutter, D. Batallas, F. Rojas-Runjaic, F. Vargas-Salinas, G. Gagliardi-Urrutia, J. C. Chaparro, L. Diaz, J. M. Padial, E. Twomey, M. Read, R. Ibáñez, P. Gaucher, A. Kwet, J. Köhler, I. De La Riva, L. F. Toledo, R. Márquez, A. García, and M. Ortega-Andrade for call recordings; Carl R. Hutter for access to his georeferenced localities database; M. Ortiz, A. Gordillo and P. I. Simões for help with bioacous- tic analysis; E. Barona, O. Cuellar, S. Duarte, A. Sánchez, M. Santana, M. C. Basto-Riascos, J. Vieira, M. Pasaje, A. Lopez- Velazquez, D. Basanta, A. F. Soto, M. G. Garcia, A. Mora, A. I. Contreras, N. Ceron, R. Murrieta, A. Montaño D. Jimenez, A. Rodriguez and Jose Criollo for assistance with field re- cordings; F. Villalobos, L. Harmon, M. Araya-Salas and Eliot Miller for assistance with phylogenetic methods; W. C. Funk, A. Cicchino, A. Gonzalez-Voyer and J. P. Jaramillo for com- ments that substantially improved this manuscript.
References Arita, H. T., Andre, J. A., Rodrıguez P., & Soberon, J. (2008). Species diversity and distribution in presence-absence matrices: Mathemat- ical relationships and biological implications. American Naturalist, 172, 519–532. https://doi.org/10.1086/590954 Ashton, K. G. (2002). Do amphibians follow Bergmann’s rule? Cana- dian Journal of Zoology, 80, 708–716. https://doi.org/10.1111/ j.1558-5646.2007.00297.x Augusto-Alves, G., Dena, S., & Toledo, L. F. (2021). Acoustic allome- try, background stream noise and its relationship with large-bodied and voiceless rheophilic frogs. Zoologischer Anzeiger, 295, 156– 162. https://doi.org/10.1016/j.jcz.2021.10.004 Bergmann, C. (1847). Über die Ver-hältnisse der wärmeokönomie der Thierezu ihrer Grösse. Göttinger Studien. Blair, C., & Doan, T. M. (2009). Patterns of community structure and microhabitat usage in Peruvian Pristimantis (Anura: Straboman- tidae). Copeia 2009:303–312. https://doi.org/10.1643/CH-08-062 Bosch, J. (2001). Female reciprocal calling in the Iberian midwife toad (Alytes cisternasii) varies with male call rate and dominant fre- quency: Implications for sexual selection. Naturwissenschaften, 88, 434–437. https://doi.org/10.1007/s001140100261 Bosch, J., & Riva, I. D. (2004). Are frog calls modulated by the envi- ronment? An analysis with anuran species from Bolivia. Canadian Journal of Zoology, 82, 880–888. https://doi.org/10.1139/z04-060 Bouckaert, R., Heled, J., Kühnert, D., Vaughan, T., Wu, C. -H., Xie, D., Suchard, M. A., Rambaut, A., & Drummond, A. J. (2014). BEAST 2: A software platform for bayesian evolutionary analysis. PLoS Computational Biology, 10, e1003537. https://doi.org/10.1371/ journal.pcbi.1003537 Bradbury, J. W., & Vehrencamp, S. L. (2012). Principles of animal com- munication, Second Edition. Sinauer Associates Inc. Brown, W. L., & Wilson, E. O. (1956). Character displacement. System- atic Zoology, 5, 49–64. Brunner, R. M., & Guayasamin, J. M. (2020). Nocturnal visual dis- plays and call description of the cascade specialist glassfrog Sachatamia orejuela. Behaviour, 157, 1257–1268. https://doi. org/10.1163/1568539X-bja10048 Bush, S. L., Gerhardt, H. C., & Schul, J. (2002). Pattern recognition and call preferences in treefrogs (Anura: Hylidae): A quantitative analysis using a no-choice paradigm. Animal Behaviour, 63, 7–14. https://doi.org/10.1006/anbe.2001.1880 Cardoso, G. C., & Price, T. D. (2010). Community convergence in bird song. Evolutionary Ecology, 24, 447–461. https://doi.org/10.1007/ s10682-009-9317-1 Carvajal-Castro, J. D., & Vargas-Salinas, F. (2016). Stream noise, habi- tat filtering, and the phenotypic and phylogenetic structure of Neo- tropical anuran assemblages. Evolutionary Ecology, 30, 451–469. https://doi.org/10.1007/s10682-016-9817-8 Castroviejo-Fisher, S., Guayasamin, J. M., Gonzalez-Voyer, A., & Vilà C. (2014). Neotropical diversification seen through glassfrogs. Jour- nal of Biogeography, 41, 66–80. https://doi.org/10.1111/jbi.12208 Chek, A. A., Bogart, J. P., & Lougheed, S. C. (2003). Mating signal partitioning in multi-species assemblages: A null model test using frogs. Ecology Letters, 6, 235–247. https://doi.org/10.1046/j.1461- 0248.2003.00420.x Chesser, R. T., & Zink, R. M. (1994). Modes of speciation in birds: A test of Lynch’s method. Evolution (N. Y), 48, 490–497. https://doi. org/10.1111/j.1558-5646.1994.tb01326.x Cooper, N., Thomas, G. H., Venditti, C., Meade, A., & Freckleton, R. P. (2016). A cautionary note on the use of Ornstein Uhlenbeck mod- els in macroevolutionary studies. Biological Journal of the Linnean Society, 118, 64–77. https://doi.org/10.1111/bij.12701 Delia, J., Bravo-Valencia, L., & Warkentin, K. M. (2017). Patterns of parental care in Neotropical glassfrogs: Fieldwork alters hypoth- eses of sex-role evolution. Journal of Evolutionary Biology, 30, 898–914. https://doi.org/10.1111/jeb.13059 Drummond, A. J., Ho, S. Y. W., Phillips, M. J., & Rambaut, A. (2006). Relaxed phylogenetics and dating with confidence. PLoS Biology, 4, e88. https://doi.org/10.1371/journal.pbio.0040088 Dugo-Cota, A., Castroviejo-Fisher, S., Vilà C., & Gonzalez-Voyer, A. (2015). A test of the integrated evolutionary speed hypothesis in a Neotropical amphibian radiation. Global Ecology and Biogeogra- phy, 24, 804–813. https://doi.org/10.1111/geb.12318 Endler, J. A. (1992). Signals, signal conditions, and the direction of evolution. American Naturalist, 139, S125–S153. https://doi. org/10.1086/285308 Escalona, M. D., Ivo Simões P., Gonzalez-Voyer, A., & Castroviejo-Fish- er, S. (2019). Neotropical frogs and mating songs: The evolution of advertisement calls in glassfrogs. Journal of Evolutionary Biology, 32, 163–176. https://doi.org/10.1111/jeb.13406 Ey, E., & Fischer, J. (2009). The “acoustic adaptation hypothesis”—a review of the evidence from birds, anurans and mammals. Bio- acoustics, 19, 21–48. https://doi.org/10.1080/09524622.2009.97 53613 Garey, M. V., Provete, D. B., Gonçalves-Souza T., Ouchi-Melo, L. S., Hadd- ad, C. F. B., & Rossa-Feres, D. C. (2018). Phylogenetic and adaptive components of the anuran advertisement call correlate with temporal species co-occurrence. Biological Journal of the Linnean Society, 125, 292–301. https://doi.org/10.1093/biolinnean/bly129 Gasc, A., Francomano, D., Dunning, J. B., & Pijanowski, B. C. (2017). Future directions for soundscape ecology: The importance of ornithological contributions. Auk, 134, 215–228. https://doi. org/10.1642/AUK-16-124.1 Gerhardt, H. C. (1994). The evolution of vocalization in frogs and toads. Annual Review of Ecology and Systematics, 25, 293–324.https://doi.org/10.1146/annurev.es.25.110194.001453 Gerhardt, H. C., & Huber, F. (2002). Acoustic communication in in- sects and anurans. Common problems and diverse solutions. Chi- cago University Press.
Gerhardt, H. C., & Mudry, K. M. (1980). Temperature effects on frequency preferences and mating call frequencies in the green treefrog, Hyla cinerea (Anura: Hylidae). Journal of Comparative Physiology, 137, 1–6. https://doi.org/10.1007/bf00656911 Gingras, B., Mohandesan, E., Boko, D., & Fitch, W. T. (2013). Phylo- genetic signal in the acoustic parameters of the advertisement calls of four clades of anurans. BMC Evolutionary Biology, 13, 1341. https://doi.org/10.1186/1471-2148-13-134 Gottsberger, B., & Gruber, E. (2004). Temporal partitioning of re- productive activity in a neotropical anuran community. Jour- nal of Tropical Ecology, 20, 271–280. https://doi.org/10.1017/ S0266467403001172 Goutte, S., Dubois, A., Howard, S. D., Marquez, R., Rowley, J. J. L., Deh- ling, J. M., Grandcolas, P., Rongchuan, X., & Legendre, F. (2016). En- vironmental constraints and call evolution in torrent-dwelling frogs. Evolution (N. Y), 70, 811–826. https://doi.org/10.1111/evo.12903 Goutte, S., Dubois, A., Howard, S. D., Márquez R., Rowley, J. J. L., Dehling, J. M., Grandcolas, P., Xiong, R. C., & Legendre, F. (2018). How the environment shapes animal signals: A test of the acoustic adaptation hypothesis in frogs. Journal of Evolutionary Biology, 31, 148–158. https://doi.org/10.1111/jeb.13210 Goutte, S., Dubois, A., & Legendre, F. (2013). The importance of am- bient sound level to characterise anuran habitat. PLoS One, 8, e78020. https://doi.org/10.1371/journal.pone.0078020 Gouveia, S. F., Dobrovolski, R., Lemes, P., Cassemiro, F. A. S., & Diniz-filho, J. A. F. (2013). Environmental steepness, tolerance gradient, and ecogeographical rules in glassfrogs (Anura: Centrol- enidae). Biological Journal of the Linnean Society, 108, 773–783. https://doi.org/10.1111/bij.12018 Grether, G. F., Losin, N., Anderson, C. N., & Okamoto, K. (2009). The role of interspecific interference competition in character displacement and the evolution of competitor recognition. Bi- ological Review, 84, 617–635. https://doi.org/10.1111/j.1469- 185x.2009.00089.x Grossenbacher, D. L., & Whittall, J. B. (2011). Increased floral diver- gence in sympatric monkeyflowers. Evolution (N. Y), 65, 2712– 2718. https://doi.org/10.1111/j.1558-5646.2011.01306.x Guayasamin, J. M., Castroviejo-fisher, S., Ayarzagüena J., Trueb, L., & Vilà C. (2008). Molecular phylogenetics and evolution phylogenet- ic relationships of glassfrogs (Centrolenidae) based on mitochon- drial and nuclear genes. Molecular Phylogenetics and Evolution, 48, 574–595. https://doi.org/10.1016/j.ympev.2008.04.012 Guayasamin, J. M., Castroviejo-Fisher, S., Trueb, L., Ayarzagüena J., Rada, M., Vilà C., Ayarzagüena J., Rada, M., & Vilà C. (2009). Phylogenetic systematics of Glassfrogs (Amphibia: Centrolenidae) and their sister taxon Allophryne ruthveni. Zootaxa, 2100, 1–97. https://doi.org/10.11646/zootaxa.2100.1.1 Guayasamin, J. M., Cisneros-Heredia, D. F., McDiarmid, R. W., Peña P., & Hutter, C. R. (2020). Glassfrogs of Ecuador: Diversity, evolution, and conservation. Diversity, 12, 222. https://doi.org/10.3390/d12060222 Guillerme, T., & Healy, K. (2014). mulTree: a package for running MCMCglmm analysis on multiple trees. Zenodo. https://doi. org/10.5281/zenodo.12902 Hadfield, J. D. (2010). MCMC methods for multi-response generalized linear mixed models: The MCMCglmm R package. J. Stat. Softw, 033, 1–22. https://doi.org/10.18637/jss.v033.i02 Hansen, T. F. (1997). Stabilizing selection and the comparative anal- ysis of adaptation. Evolution (N. Y), 51, 1341–1351. https://doi. org/10.1111/j.1558-5646.1997.tb01457.x Harmon, L. J., Andreazzi, C. S., Débarre F., Drury, J., Goldberg, E. E., Martins, A. B., Melián C. J., Narwani, A., Nuismer, S. L., Pennell, M. W., Rudman, S. M., Seehausen, O., Silvestro, D., Weber, M., & Matthews, B. (2019). Detecting the macroevolutionary signal of species interactions. Journal of Evolutionary Biology, 32, 769–782. Harmon, L. J., Weir, J. T., Brock, C. D., Glor, R. E., & Challenger, W. (2007). GEIGER: Investigating evolutionary radiations. Bioinfor- matics, 24, 129–131. https://doi.org/10.1093/bioinformatics/btm538 Höbel, G., & Gerhardt, H. C. (2003). Reproductive character dis- placement in the acoustic communication system of green tree frogs (Hyla cinerea). Evolution (N. Y), 57, 894–904. https://doi. org/10.1111/j.0014-3820.2003.tb00300.x Humfeld, S. C., & Grunert, B. (2015). Effects of temperature on spec- tral preferences of female gray treefrogs (Hyla versicolor). Herpe- tological Conservation and Biology, 10, 1013–1020. Hutter, C. R., & Guayasamin, J. M. (2012). A new cryptic species of glass- frog (Centrolenidae: Nymphargus) from Reserva Las Gralarias, Ecuador, Zootaxa, 21, 1–21. https://doi.org/10.11646/ZOOTAXA.3257.1.1 Hutter, C. R., Guayasamin, J. M., & Wiens, J. J. (2013). Explaining Andean megadiversity: The evolutionary and ecological causes of glassfrog elevational richness patterns. Ecology Letters, 16, 1135– 1144. https://doi.org/10.1111/ele.12148 Indermaur, L., Schmidt, B. R., Tockner, K., & Schaub, M. (2010). Spa- tial variation in abiotic and biotic factors in a floodplain determine anuran body size and growth rate at metamorphosis. Oecologia, 163, 637–649. https://doi.org/10.1007/s00442-010-1586-4 Jansen, M., Plath, M., Brusquetti, F., & Ryan, M. J. (2016). Asymmetric fre- quency shift in advertisement calls of sympatric frogs. Amphibia-Reptil- ia, 37, 137–152. https://doi.org/10.1163/15685381-00003038 Karger, D. N., & Zimmermann, N. E. (2019). Climatologies at high res- olution for the earth land surface areas CHELSA V1. 2: Technical specification. Swiss Fed. Res. Inst. WSL, Switz. Klymus, K. E., Humfeld, S. C., & Gerhardt, H. C. (2012). Geographical variation in male advertisement calls and female preference of the wide-ranging canyon treefrog, Hyla arenicolor. Biological Journal of the Linnean Society, 107, 219–232. https://doi.org/10.1111/ j.1095-8312.2012.01931.x Köhler, J., Jansen, M., Rodríguez A., Kok, P. J. R., Toledo, L. F., Emmrich, M., Glaw, F., Haddad, C. F. B., Rödel M. O., & Venc- es, M. (2017). The use of bioacoustics in anuran taxonomy: Theory, terminology, methods and recommendations for best practice. Zootaxa, 4251, 1–124. https://doi.org/10.11646/zoo- taxa.4251.1.1 Krause, B. L. (1993). The niche hypothesis: A virtual symphony of an- imal sounds, the origins of musical expression and the health of habitats. Soundscape Newsl., 6, 6–10. Kumar, S., Stecher, G., & Tamura, K. (2016). MEGA7: Molecular evolu- tionary genetics analysis version 7.0 for bigger datasets. Molecular Biology and Evolution, 33, 1870–1874. https://doi.org/10.1093/ molbev/msw054 Laliberté, E., & Legendre, P. (2010). A distance-based framework for measuring functional diversity from multiple traits. Ecology, 91, 299–305. https://doi.org/10.1890/08-2244.1 Lanfear, R., Frandsen, P. B., Wright, A. M., Senfeld, T., & Calcott, B. (2016). PartitionFinder 2: New methods for selecting partitioned models of evolution for molecular and morphological phylogenetic analyses. Molecular Biology and Evolution, 34, 772–773. https:// doi.org/10.1093/molbev/msw260 Lemmon, E. M. (2009). Diversification of conspecific signals in sym- patry: Geographic overlap drives multidimensional reproductive character displacement in frogs. Evolution (N. Y), 63, 1155–1170. https://doi.org/10.1111/j.1558-5646.2009.00650.x Liu, H. Q., & Huete, A. (1995). A feedback based modification of the NDVI to minimize canopy background and atmospheric noise. IEEE Transactions on Geoscience and Remote Sensing, 33, 457– 465. https://doi.org/10.1109/TGRS.1995.8746027 Losos, J. B., & Glor, R. E. (2003). Phylogenetic comparative methods and the geography of speciation. Trends in Ecology and Evolution, 18, 220–227. https://doi.org/10.1016/S0169-5347(03)00037-5 Marquet, P. A., Fernández, M., Navarrete, S. A. & Valdovinos, C. (2004). Diversity emerging: Towards a deconstruction of biodi- versity patterns. In M. Lomolino & L. Heansey (Eds.), Frontiers in biogeography: New directions in the geography of nature (pp. 191–209). Sinauer Associates, Inc. Marten, K., & Marler, P. (1977). Sound transmission and its signifi- cance for animal vocalization. Behavior, Ecology and Sociobiology, 2, 271–290. https://doi.org/10.1007/bf00299740 Mayfield, M. M., & Levine, J. M. (2010). Opposing effects of com- petitive exclusion on the phylogenetic structure of communities.
Ecology Letters, 13, 1085–1093. https://doi.org/10.1111/j.1461-0248.2010.01509.x Mayr, E. (1942). Systematics and the origin of species. Columbia Uni- versity Press. Meenderink, S. W. F., Kits, M., & Narins, P. M. (2010). Frequency matching of vocalizations to inner-ear sensitivity along an altitudi- nal gradient in the coqui frog. Biology Letters, 6, 278–281. https:// doi.org/10.1098/rsbl.2009.0763 Meier, J. I., Marques, D. A., Mwaiko, S., Wagner, C. E., Excoffier, L., & Seehausen, O. (2017). Ancient hybridization fuels rapid cichlid fish adaptive radiations. Nature Communications, 8, 1–11. https://doi. org/10.1038/ncomms14363 Mendoza, A. M., & Arita, H. T. (2014). Priority setting by sites and by species using rarity, richness and phylogenetic diversity: The case of neotropical glassfrogs (Anura: Centrolenidae). Biodiversity and Con- servation, 23, 909–926. https://doi.org/10.1007/s10531-014-0642-5 Mendoza, A. M., Bolívar-García W., Vázquez-Domínguez E., Ibáñez R., & Parra Olea, G. (2019). The role of Central American barriers in shaping the evolutionary history of the northernmost glassfrog, Hyalinobatrachium fleischmanni (Anura: Centrolenidae). PeerJ, 7, e6115. https://doi.org/10.7717/peerj.6115 Mendoza-Henao, A. M., Arias, E., Townsend, J. H., & Parra-Olea, G. (2020). Phylogeny-based species delimitation and integrative tax- onomic revision of the Hyalinobatrachium fleischmanni species complex, with resurrection of H. viridissimum (Taylor, 1942). Sys- tematics and Biodiversity, 18, 464–484. https://doi.org/10.1080/14 772000.2020.1776781 Mendoza-Henao, A. M., Duarte-Marín S., & Rada, M. (2021). Adver- tisement calls of six glassfrog species in the Colombian Andes, and comments on priorities for future research and conservation. Am- phibian and Reptile Conservation, 15, 156–171. Miller, E. T., Farine, D. R., & Trisos, C. H. (2017). Phylogenetic com- munity structure metrics and null models: A review with new methods and software. Ecography (Cop.), 40, 461–477. https://doi. org/10.1111/ecog.02070 Miller, M. A., W. Pfeiffer, and T. Schwartz. (2010). Creating the CIPRES Science Gateway for inference of large phylogenetic trees. In 2010 Gateway Computing Environments Workshop (GCE) (pp. 1–8). https://doi.org/10.1109/GCE.2010.5676129 Mohneke, R., & Schneider, H. (1979). Effect of temperature upon auditory thresholds in two anuran species, Bombina v. variegata and Alytes o. obstetricans (Amphibia, Discoglossidae). Journal of Comparative Physiology, 130, 9–16. https://doi.org/10.1007/bf02582969 Narins, P. M., & Meenderink, S. W. F. (2014). Climate change and frog calls: long-term correlations along a tropical altitudinal gra- dient. Proceedings of the Royal Society B: Biological Sciences, 281, 20140401. https://doi.org/10.1098/rspb.2014.0401 Peterson, A. T., Navarro-Sigüenza, A. G., & Gordillo, A. (2016). As- sumption-versus data-based approaches to summarizing spe- cies’ ranges. Conservation Biology, 32, 568–575. https://doi. org/10.1111/cobi.12801 Pfennig, K. S., & Pfennig, D. W. (2009). Character displacement: Eco- logical and reproductive responses to a common evolutionary problem. Quarterly Review of Biology, 84, 253–276. https://doi. org/10.1086/605079 Plummer, M., Best, N., Cowles, K., & Vines, K. (2006). CODA: Conver- gence diagnosis and output analysis for MCMC. R News, 6, 7–11. Revell, L. J. (2009). Size-correction and principal components for in- terspecific comparative studies. Evolution (N. Y), 63, 3258–3268. https://doi.org/10.1111/j.1558-5646.2009.00804.x Revell, L. J. (2012). phytools: An R package for phylogenetic compara- tive biology (and other things). Methods in Ecology and Evolution, 3, 217–223. https://doi.org/10.1111/j.2041-210X.2011.00169.x Ryan, M. J., & Keddy-Hector, A. (1992). Directional patterns of female mate choice and the role of sensory biases. American Naturalist, 139, S4–S35. https://doi.org/10.1086/285303 Salgado, A. L., & Guayasamin, J. M. (2018). Parental care and repro- ductive behavior of the minute dappled glassfrog (Centrolenidae: Centrolene peristictum). South American Journal of Herpetology, 13, 211–219. https://doi.org/10.2994/SAJH-D-17-00066.1 Seddon, N., & Tobias, J. A. (2010). Character displacement from the re- ceiver’s perspective: Species and mate recognition despite convergent signals in suboscine birds. Proceedings of the Royal Society B: Biologi- cal Sciences, 277, 2475–2483. https://doi.org/10.1098/rspb.2010.0210 Stuart, Y. E., & Losos, J. B. (2013). Ecological character displacement: Glass half full or half empty? Trends in Ecology and Evolution, 28, 402–408. https://doi.org/10.1016/j.tree.2013.02.014 Thompson, J. D., Higgins, D. G., & Gibson, T. J. (1994). CLUSTAL W: Improving the sensitivity of progressive multiple sequence align- ment through sequence weighting, position-specific gap penalties and weight matrix choice. Nucleic Acids Research, 22, 4673–4680. https://doi.org/10.1093/nar/22.22.4673 Tobias, J. A., Aben, J., Brumfield, R. T., Derryberry, E. P., Halfwerk, W., Slabbekoorn, H., & Seddon, N. (2010). Song divergence by sen- sory drive in Amazonian birds. Evolution (N. Y), 64, 2820–2839. https://doi.org/10.1111/j.1558-5646.2010.01067.x Tobias, J. A., Cornwallis, C. K., Derryberry, E. P., Claramunt, S., Brum- field, R. T., & Seddon, N. (2014a). Species coexistence and the dy- namics of phenotypic evolution in adaptive radiation. Nature, 506, 359–363. https://doi.org/10.1038/nature12874 Tobias, J. A., Planqué R., Cram, D. L., Seddon, N., Planque, R., Cram, D. L., & Seddon, N. (2014b). Species interactions and the structure of complex communication networks. Proceedings of the National Academy of Sciences of the United States of America, 111, 1020– 1025. https://doi.org/10.1073/pnas.131433711 Tonini, J. F. R., Provete, D. B., Maciel, N. M., Morais, A. R., Goutte, S., Toledo, L. F., & Pyron, R. A. (2020). Allometric escape from acoustic constraints is rare for frog calls. Ecology and Evolution, 10, 3686–3695. https://doi.org/10.1002/ece3.6155 Twomey, E., Delia, J., & Castroviejo-fisher, S. (2014). A review of Northern Peruvian glassfrogs (Centrolenidae), with the description of four new remarkable species. Zootaxa, 3851, 1–87. https://doi. org/10.11646/zootaxa.3851.1.1 Villanueva-Rivera, L. J. (2014). Eleutherodactylus frogs show frequen- cy but no temporal partitioning: Implications for the acoustic niche hypothesis. PeerJ, 2, e496. https://doi.org/10.7717/peerj.496 Webb, C. O., Ackerly, D. D., McPeek, M. A., & Donoghue, M. J. (2002). Phylogenies and community ecology. Annual Review of Ecology and Systematics, 33, 475–505. https://doi.org/10.1146/annurev. ecolsys.33.010802.150448 Wellborn, G. A., Skelly, D. K., & Werner, E. E. (1996). Mechanisms creating community structure across a freshwater habitat gradient. Annual Review of Ecology and Systematics, 27, 337–363. https:// doi.org/10.1146/annurev.ecolsys.27.1.33 Wells, K. D., & Schwartz, J. J. (2007). The behavioral ecology of anu- ran communication. In P. M. Narins, A. S. Feng, R. R. Fay & A. N. Popper (Eds.), Hearing and sound communication in amphibians (pp. 44–86). Springer. West-Eberhard, M. J. (1983). Sexual selection, social competition, and speciation. Quarterly Review of Biology, 58, 155–183. Wiley, R. H. (1991). Associations of song properties with habitats for territorial Oscine birds of Eastern North America. American Natu- ralist, 138, 973–993. https://doi.org/10.1086/285263 Wiley, R. H., & Richards, D. G. (1982). Adaptations for acoustic com- munication in birds: Sound transmission and signal detection. In D. Kroodsma & E. Miller (Eds.), Acoustic communication in birds (pp. 131–181). Academic Press. Wilkins, M. R., Seddon, N., & Safran, R. J. (2013). Evolutionary divergence in acoustic signals: Causes and consequences. Trends in Ecology and Evolution, 28, 156–166. https://doi.org/10.1016/j.tree.2012.10.002 Ziegler, L., Arim, M., & Bozinovic, F. (2016). Intraspecific scaling in frog calls: The interplay of temperature, body size and metabolic condition. Oecologia, 181, 673–681. https://doi.org/10.1007/s00442-015-3499-8 Ziegler, L., Arim, M., & Narins, P. M. (2011). Linking amphibian call structure to the environment: The interplay between phenotypic flexibility and individual attributes. Behavioral Ecology, 22, 520– 526. https://doi.org/10.1093/beheco/arr011 Zimmerman, B. L. (1983). A comparison of structural features of calls of open and forest habitat frog species in the central Amazon. Her- petologica, 39, 235–246.
Kommentare